Speaker Details
Tutorial
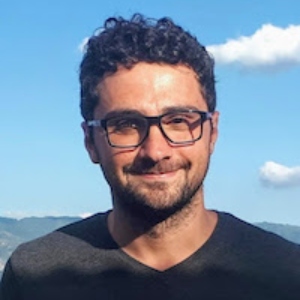
Ludovik Coba
Ludovik Coba holds a PhD in Computer Science from the Free University of Bozen-Bolzano. He is currently a Machine Learning Scientist at Expedia Group working on innovating recommender systems for the travel industry. He publishes his research in venues like RecSys or IUI and journals like IT and Tourism, IEEE Computational Intelligence Magazine. He also served on the organising committee of conferences like RecSys and UMAP and as a PC for IAAA, TheWebConf, and more.
Tutorial title: Interpretability of Machine Learning Models
Abstract:
Machine learning (ML) is being adopted across various fields, including e-commerce, healthcare, finance, autonomous vehicles, manufacturing, energy, entertainment, cybersecurity, and more. Its applications range from personalized recommendations to medical diagnosis, transforming industries and improving decision-making processes across society. As ML becomes more entangled with our lifestyles, it becomes important to explain these models. By providing insights into the reasoning behind model predictions, explainability improves stakeholders’ confidence and facilitates a better understanding of how the model functions. Furthermore, it ensures compliance with regulations in industries where accountability and ethical considerations are paramount, such as healthcare and finance. However, explainability is not easy. To make things more complicated, there is no universal definition of explainability, and the requirements may vary depending on the application and stakeholders. In this tutorial, I will introduce the problems and challenges of ML interpretability. I will present post-hoc explanation approaches, which, often, are considered the answer to explaining most ML models, and I will show how we can fool them. Finally, I will discuss the problem of evaluating explainability.